Explainable AI Technical Whiteboard Series: SHAP
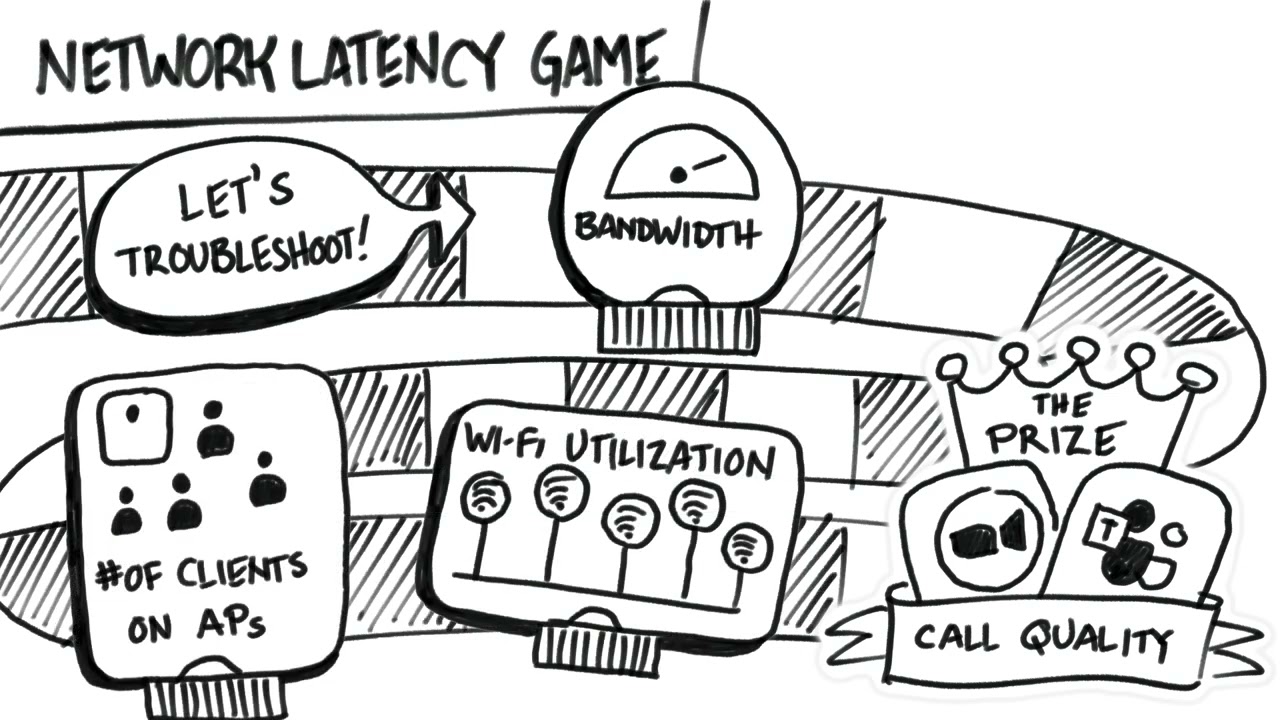
Technical Whiteboard Series: SHAP
Learn how Shapley Additive Explanations (SHAP) enhances trust and integration of machine learning solutions. We include practical networking examples, such as an explanation of video conferencing latency predictions for Zoom and Microsoft Teams. Join us to understand how SHAP makes AI more transparent and trustworthy, leading to better user and operator experiences.
You’ll learn
What SHAP is
How SHAP values provide transparency in AI models
Applications of SHAP in network latency troubleshooting
Who is this for?
Experience More
Transcript
0:06 today we look at explainable Ai and a
0:08 technique called shap which is short for
0:11 shly additive
0:13 explanations AI models don't natively
0:15 explain themselves so data science teams
0:18 must rely on tools and techniques to
0:20 help users understand how the AI Works
0:23 in this video we explore shap one such
0:26 technique used to build trust and
0:28 confidence shap makes machine learning
0:30 models transparent by providing detailed
0:33 visual explanation of the model's
0:35 predictions and an equal initial waiting
0:37 for all model parameters with
0:39 interpretable insights of machine
0:41 learning model output shap helps
0:43 operators to understand and Trust what
0:45 they're using this leads to better
0:48 acceptance and integration of machine
0:49 learning Solutions in various
0:52 applications shapley values were
0:54 introduced in 1951 by Lloyd chapley
0:58 using Concepts from Cooperative game
1:00 Theory it assigns an importance value
1:02 for features or players for a particular
1:04 prediction all features are treated
1:06 equally as input to the
1:08 calculation in a game of network latency
1:10 troubleshooting the players are features
1:12 like bandwidth Wi-Fi radio utilization
1:15 number of clients on an access point use
1:17 of vpns Etc shap is used to assign a
1:21 value to each feature that represents
1:23 how much that feature contributed to the
1:25 final prediction based on every
1:27 combination of possible features by
1:29 looking at all combinations all features
1:31 are fairly represented and features that
1:33 are dependent or synergistic are modeled
1:36 for a given prediction shap values
1:38 explain the impact of each feature
1:40 included in the model which provides
1:42 transparency waiting and The elusive
1:44 answers to the Y
1:46 questions shap values can be visualized
1:49 in various ways such as bar charts for
1:51 individual predictions or summary plots
1:54 for overall feature importance shap
1:56 provides a detailed quantifiable and
1:59 understandable way to explain how each
2:01 feature in a data set influences a
2:03 model's predictions shap values are
2:05 based on data and are unbiased we can
2:08 validate and gain confidence about shap
2:10 values when it reinforces Network domain
2:13 knowledge for example a high Wii radio
2:16 utilization is a clear sign of a Wi-Fi
2:19 network reaching capacity if the shap
2:21 value is large for the radio utilization
2:23 feature when the AP has high radio usage
2:26 we know the model has correctly learned
2:28 the system this makes it a powerful tool
2:31 for enhancing transparency and
2:32 explainability in ai explainable ai is
2:36 closely related to
2:38 interpretability interpretability gives
2:40 context to the meaning of an AI model's
2:42 output many platforms already interpret
2:45 outputs but few of them provide a
2:47 measure of explanation behind those
2:49 interpretations at Juniper Networks shap
2:52 helps to explain video conferencing
2:54 latency predictions for zoom and
2:56 Microsoft teams Juniper employs deep
2:58 learning models that are trained on
3:00 incredibly large data sets and shap
3:02 helps to explain the model's predictions
3:04 so operators can rapidly troubleshoot
3:06 and prioritize their efforts this
3:09 ensures not just exceptional user
3:11 experiences but also the best operator
3:13 experience at Juniper our AI native
3:16 design philosophy focuses on making
3:18 every connection count