EP. 1 AI for IT: Accelerating Network Deployment and Improving User Experience

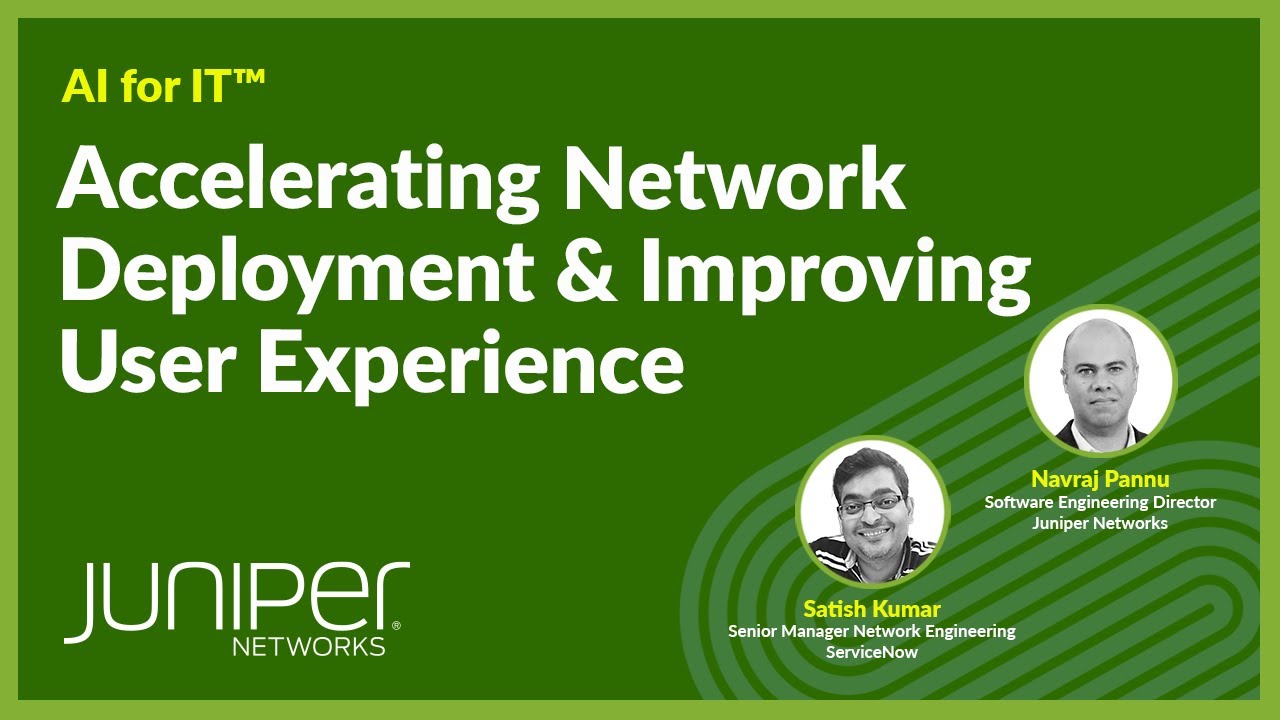
With Juniper Mist AI, ServiceNow has the ultimate lens into user experiences.
Do you know the difference between traditional opaque AI and truly explainable AI? Watch this webinar for answers, as well as a tour of Juniper Mist AI™ capabilities, how its machine algorithms work, and how ServiceNow has benefited.
Ready to take the next step to enhance your network while cutting costs? Check out our live demo to see how Juniper Mist AI can help you enhance user experiences, improve productivity, and increase scalability. By signing up, you could qualify for a free AP and trial of Mist!
You’ll learn
How ServiceNow proactively identifies and fixes Wi-Fi problems
How partnering with Juniper helped ServiceNow improve Zoom quality
Who is this for?
Host

Guest speakers


Experience More
Transcript
0:07 we're going to go ahead and get started um thank you again for joining we are so excited to have you all in attendance today um before we kick things off I do
0:14 want to take some time to introduce our speakers uh today we have Satish Kumar who is a senior network manager at
0:21 service now as well as navraj panu who's the director of data science here at
0:27 Juniper um and my name is Selena gatis uh I am an AI Ops uh pmm here at Juniper
0:33 and I'll be the moderator for today's session um so jumping right in during this webinar we'll talk about an
0:40 impactful AI powered feature that was developed with the help of our friends at service now we'll show you what's
0:45 behind the AI curtain exposing how AI Works which is also known as explainable Ai and how Juniper developed this AI
0:53 capability to improve the experience of something most organizations use today and coincidentally what we're using
0:58 today to host our webinar um zoom and then finally we'll have some time at the end for a brief Q&A so please place them
1:06 into the Q&A section throughout the webinar um and then also during the
1:11 presentation we'll do some fun trivia questions just to test your AI history knowledge um so let's go ahead and get
1:18 right started with understanding Ai and networking uh Na Raj I'm gonna direct
1:23 this first question to you uh as a data scientist can you describe your dayto
1:28 day and can you please share what goes into building an AI sure um I guess the first thing that
1:36 comes to mind is we need a question we need a question to answer so for example in this
1:43 particular uh topic my question was can you predict if your Zoom call will be
1:50 good right so it's quite a general question then we have to see if we can
1:55 find tune it and have data in order to train the model so how did we fine-tune
2:00 that question we asked can we predict the latency of a zoom call or can we
2:07 predict how many times a network packet loss will happen during the zoom call so
2:12 that's something that's measurable and we can then Define a metric to Def to see how well our model is doing for
2:19 example can our model predict the latency of the zoom call right and the
2:25 nice thing is is that um Zoom provides us this inform information so we have
2:31 labels Zoom provides us with the latency for um the audio video and also if there
2:38 was packet losses so then we can develop our model and see how well it agrees so
2:44 we have our first metric how close are we are we within can we predict to
2:50 within for example 20 milliseconds uh the latency for 90% of the calls right and that that's actually
2:57 the metric that was uh agreed by the PMs and that's what we did and we succeeded
3:04 right so then the next question is as you asked how do you actually develop this model well you have to think about
3:11 all of the important things that go into the model and if we have the data the nice thing is we had the data from zoom
3:17 and we have our Juniper Miss data all in the cloud so we can combine them so what were some of the features or network
3:25 parameters that we use in our model well one thing is are we too is a client too
3:31 far away from the access point right that's a measure of the Wi-Fi signal if it's poor chances are or you might have
3:39 a poor experience are there too many clients on a particular access point is
3:44 it too crowded does that affected all of these are open questions no one knows right no one knows the answer these
3:50 questions and that's one of the exciting things about developing a model we can answer these things or you know for
3:56 example is it um a site problem is you know just there not enough bandwidth or latency at the site level that is again
4:04 another Network parameter that we use so we take into account all of these features or network parameters that we
4:11 have we have tons of data millions of data points for that and then we can develop a model so what model do we use
4:18 you know we've heard about chat GPT and you know some of the Specialists might have heard about Transformers or neural
4:24 networks or tree based methods you know those are all open to us and the nice thing about data science scientist
4:30 that's where the science comes in you experiment you see what is best you look at how much data that you have for
4:36 example if you have millions you can have a very complex model so some people
4:41 might have heard how long it takes to train chat GPT like a month uh on you know a dedicated cluster of gpus right
4:49 they have tons of data you know our data is a bit less but you know still it's a significant amount of data if you look
4:55 at the Miss Universe so we can develop very complex models so and that's in fact what we did and always the hard
5:02 part when you're developing a model the hard part is getting the data one of the hard Parts the second is is it actually
5:08 working and that's where you know predefining the metric for example how close we are to the
5:14 latency that is an indication of its performance and if it's performing well
5:19 that's great and we have a good indication about going forward with it
5:24 but we go back to the question can you predict if the
5:30 uh if my network will support an audio uh call and can you explain what could
5:37 be the problem and that was a big uh issue here can we actually explain what the problem is so for example was a
5:44 client too far away from the access point so that's the second part once we have a model and that we can trust it we
5:51 go to the explainability there's many different algorithms also to do this so
5:56 there's for example you can check out search lime uh and explainability AI
6:04 there's you know you can remove one of these Network parameters at one at a time and see the effect it has on the
6:10 model the one that we had chosen to do is called chapley it's a industry standard used um quite a bit in for
6:18 example the fraud industry trying to determine what are the parameters for fraud and um also in any many different
6:26 other types of predictions so with this shoply then we can see what is the
6:32 dominant reason for our call failing so was it a client problem it could have
6:38 been the particular um model for example device that a client was using that's that's a
6:46 possible reason maybe there was a client um configuration that was used that led
6:52 to this problem as long as it's a network parameter in our model we'd be able to predict it or for example if
6:58 there was just not enough latency at the site level to do that so when all of
7:03 that is done and validated and we go through quite a different uh quite an
7:08 extensive validation process at Juniper where the project managers come in and luckily we also had Satish come in and
7:16 say you know this H this makes sense so which is uh anytime we can get dedicated
7:22 customer uh critical feedback it's it's gold so and then uh once we pass that
7:29 we push it out to production and also equally good I work with great colleagues who can scale up this um the
7:38 models that we've developed make it generally available it's awesome sounds like tons
7:44 and tons of work that goes into it none enough words in the world can explain it huh
7:49 um so let's before we jump right on into our next session here uh let's run a
7:54 quick poll or trivia question if you will um I will go ahead head and put
8:00 that up the one was the concept of artificial intelligence first
8:06 coed I'll give a few seconds here see some answers coming
8:19 in hey
8:24 so all right so if you answered we can go over to the next
8:32 slide awesome if you answered 1956 you are correct so gold star for
8:38 you um great job let's move on over to our next
8:44 section here uh so fatish let's let's talk about the AI
8:51 solution benefits for service now um so s why did you choose Juniper and what
8:57 are some benefits you've achieved using our AI solution absolutely um let me start
9:05 about maybe about five years ago and service now we started with M Wireless
9:11 then it was working fine great right we saw a lot of the wireless issues what we
9:17 had before Miss migration then about two years ago uh
9:22 when the jiper acquired Mist right that's where we we we started thinking of um uh moving toward the switching and
9:30 also the Juniper SSR which is a 128 company which is acquired by Juniper
9:36 right so two years ago we started with this migrating to switching then SSR so
9:42 as a last year we are like a full full stack Juniper uh Wireless wired and van
9:49 so why did we choose Juniper right so we have mainly I'll put it in the three
9:54 ways right so the four four reasons mainly one is simplify the network
9:59 operations and second one is bring the end to end visibility
10:05 and the third one is bring the better user experience and the last one is
10:10 obviously the cost Factor right so if you look at the first one the simplified network operations what I mean by that
10:18 uh before Juniper it was very challenging uh for a network team to do the operations right either the code
10:24 upgrades or the board configuration or vand changes right all the day to operations it was never easy and
10:32 automation capabilities is also very limited so that is where the Juniper platform helped us to simplify right how
10:39 did it simplify so it is easy to um provision right using a ztp zero touch
10:46 provisioning is like a very good one right it's we never had before that like that I always call it as a true ztp it's
10:54 a cloud-based just like a scan barcode and all it need is a d CP IP address
10:59 connectivity and rest everything is pushed from the Mist right so it simplified overall provisioning process
11:07 even bringing up new sites it was never easy before so now we don't do any travels much so we pretty much do all
11:14 the uh the work done remotely by the network Engineers having maybe local
11:19 contractor do the raken stack physical work things like that right so that's on the uh Day Zero part
11:27 and also there's another one important U which is the templates which is I would say this is like a great great
11:34 capability we always had a challenge on maintaining the network configuration
11:39 standard right it's easy I mean any network engineer they go through that we
11:45 Define the standard in a spreadsheet but maintaining the spreadsheet is never easy and it'll get lost over the period
11:51 of time so what we Define in the spreadsheet and uh it's never be the same on the um on the actual Network
11:59 devices so this template is really simplified that and we also have the
12:04 compliance compliance we need to make sure we follow certain guidelines on the configuration right so the templates it
12:12 greatly not only simplified and it also helped us to maintain the network
12:17 configuration standards throughout the life cycle so now I can tell confidently
12:23 without even logging to any device by by going to the templates I can tell hey what is our standard and U the Mist
12:31 takes take care of all the magic right we don't have to log into devices we don't have to do any scanning everything
12:37 everything is techn about it so that is another second good feature and the third one the next one is a dtp dynamic
12:45 uh Port profiling it so this is also a great feature because most of most of
12:52 the operations work involved configuring the ports things like that I mean the new devices comes and goes away right we
12:58 got always the help team comes to say can you configure I I got a new printer
13:04 or I got a new security camera or I connected in this so support can you configure the vand so all that work is
13:11 been taken taken off now by the dynamic Port profiling how we can do the
13:17 profiling in the Mist portal so then it becomes like a more of the plugin playing right you once you do the
13:23 profiling for the devices so they can connect the help team or the support teams they can go back and they can go
13:30 to the IDF and connect the devices and this automatically configur the port without even uh network engineer to do
13:37 anything right it it works just like a magic so some of these features help to
13:42 simplify uh in the network operations right this is by by default by the mist
13:48 and at service now even we took a little bit further a step right even
13:54 um for example the uh the firware upates things like that it was super easy like
14:00 two clicks select the firmware upgrade the version then second click upgrade
14:05 and this everything is taken care by the uh the cloud right M Cloud so service
14:10 now even we have taken for the next level we have workflow this entire
14:15 process end to end uh automation workflow for the firware upgrades that
14:22 includes the validation part so now we don't do any manual upgrades we we don't even do
14:30 no including the validation is like a full end to end automation workplace it's been working great how this is all
14:37 possible because of the good Rich apis by the supported by the mist and having
14:43 the integration between two platforms to Great platforms we're able to do some of this great
14:49 stuff so another other important f is the visibility right that was another
14:54 also a very key so we always have a problem in u when things break troubleshooting is
15:02 always challenging it was never easy so we wanted we always uh wanted have good
15:07 visibility on the data that was a missing piece so when we decided to go
15:13 with the full stack Juniper so that helped to solve the problem so now we are since we are full stack Wireless
15:19 wired and Van we have full visibility in the entire network the packet goes from
15:24 the wireless wire and the van Network and having this capability of junifer
15:31 the marus missed so it can on top of that on top of the network you have the
15:36 a capabilities which is a marage so it is not only telling up down the switch
15:42 down things like that it does beyond to right it kind of predicts the network it it gives a deep visibility and it gives
15:50 the the Marv actions whether it's a DNS issues or DHCP issues or the
15:55 misconfiguration so some of those all the stuff is is out of the box right we don't have to even look into that so
16:02 overall troubleshooting has become very easy and bringing this the end to end
16:08 the visibility the data is what helping a lot and combining all these features
16:14 is what it is driving for the better user experience we want to give the
16:19 users the best um Network experience right even when there's a problem so
16:25 users need to know immediately right we're trying to move away from a reactive approach more of like a
16:33 proactive right we're trying to give some of the enable some of the features like a self service users themselves
16:40 they can troubleshoot the issues by themselves by having this having the integration between service now and the
16:48 jiper missed so we we can L some of those capabilities and let the users
16:53 troubleshoot the issues by themselves before even they reach out to their help do things like that
16:59 so yeah combining all this that's how um these are some of the reasons why we picked Juniper and uh this is where we
17:07 are this journey to that is awesome so happy to hear that we are we've checked
17:12 all the boxes and we continue checking them um naaj I don't know if you wanted to say anything before I jump right on
17:18 into our next trivia question or we can wait until next H yeah we can wait until
17:24 the next okay um so our next trivia question
17:30 here let me go ahead and put that on the screen uh what is the primary objective
17:35 of reinforcement learning in AI I'll give it a few seconds
17:57 here all righty looks like people are changing their minds putting one thing
18:03 so I'm gonna go ahead and end it here's some results if you want to
18:09 move over to the next slide if you answered learning from trial and error
18:14 you are correct so another gold star for
18:20 you um let's keep going so we're going to move over to this next section which is
18:27 sort of like the meat and potato of this webinar um be a two-part question I'll
18:33 ask something to Sati and then nav feel free to like chime in wherever um so Satish uh tell us some challenges you've
18:40 had with your Zoom calls and then navage you know how did you develop this integration to resolve those challenges
18:47 so Sati I'll go ahead and give you the floor y so service now as Zoom is always the
18:55 the most critical application the service that heavily been used right if Zoom is not
19:01 working it's it's kind of really not a great thing right so maintaining um the
19:08 zoom application all the time uh healthy and make giving the best user experience out the zoom calls is super critical for
19:16 us so even with the juper when we acquired the full stack juper right we
19:21 always had this challenges with zoom complaints so users always complain that
19:27 um they been having issues with the slowness audio video choppy all all that
19:32 kind of issues right but when we troubleshoot these kind of issues it's
19:38 very hard right even with what whatever the data we have um it it tells okay
19:44 it's not a network problem but it doesn't tell enough that uh why it is a
19:50 problem with a zoom right so that that was a missing piece so our focus is not
19:56 just passing the ball even we trying to to okay even if it is not Network issue we wanted to tell where the potential
20:02 problem area could be right so that is where then we started discussions with the Juniper Miss team and we told them
20:09 okay let's do the integration with the zoom and bring the zoom data into M
20:16 Cloud right M portal so when we do this when when that integration happened so
20:22 now we can see the zoom statistics of all the users of all the calls in the same M portal so what does it mean so
20:30 now even when somebody reports an issue it's kind of very easy um to identify
20:35 the issue and correlate whether it is because of the network issue Wireless
20:41 wide or V or is it more on the application side with is which is a
20:46 zoom so and it helps with the the correlation too and most of the time
20:52 it's what based on our experience 80 90% of the time the issues relies on the
20:58 client side right the laptop side laptops maybe High CPU or high memory
21:03 the resources running very high on the laptop those are the contributing
21:09 factors for the bad uh Zoom call experience so with with having that Zoom
21:16 integration with the McLoud bringing that data into the same one place with
21:22 the correlation helps uh so much easy for troubleshooting to give an example
21:29 we had a couple of months ago in one of the site in us um it was in Orlando right so users used
21:36 to complaint the zoom quality issues but after done the thorough troubleshooting
21:42 we found out it was due to some hardware issues and also the Wi-Fi interference
21:47 and height of the aps so then um we identified and we fix
21:53 the problem then how do we measure whether did we really fix the issues or not not right after the upgrade after
22:00 adjusting the height of the access point all the stuff so issues got settled down
22:06 right so issues automatically significantly drop right no not much
22:11 complaints on that then we wanted to see by ourself hey is it really improved or
22:17 not right that's where even the the Jer Miss team help using the AI ml algorithm
22:24 the back end they were able to provide some of the stats that tells how the experience was before the changes and
22:32 how the experience was after the changes so we are able to uh um see almost a 40%
22:39 Improvement in the zoom call overall experience based on the data that's been
22:44 provided by the M IML
22:49 team yeah and this was a a great uh example where
22:57 you know the customer and Juniper Miss got together service now Juniper Miss got together
23:03 and tried to solve this problem like and again you highlighted some very important things the whole idea was
23:09 trying to identify the problem right you know is it a client problem is it a
23:15 problem with the access points or is it a site problem you know these the these are all very sensitive issues and you
23:22 know everyone wants sometimes maybe wants to point the finger at everyone someone else but you know and that's
23:28 nice thing about the transparency about the explainable AI right so I mentioned the shle values beforehand so these you
23:36 know this is not something that we uh manually code in and say oh you know it's always a client problem or
23:42 something like that it it shows us uh based on you know it's mathematically proven even given a Nobel Prize for uh
23:50 the fact that it um uh gives a fair unbiased and objective
23:56 um contribution to whichever one of those factors are so and uh the the thing
24:03 about networks as well it's very Dynamic so things can change and they do change for example after your after the
24:10 successful upgrade right we we see the performance going on but the nice thing is we have this measure you know coming
24:17 from Zoom about the latency or the packet loss and then we can measure to
24:23 see oh did something happen next week uh or something happened last weekend now
24:28 we have a huge performance uh decrease so uh just just as another example um we
24:36 had noticed that um Zoom performance was significantly uh poor all across service
24:42 now so I I approached uh the PM um Kumar
24:48 putas Swami and I said you know what's happening so I think Kumar talked into you sa and he said oh there was an email
24:56 that um people are going back to work so and we saw that immediately so you can
25:02 see it and then you know that and just as Sati was pointing out you know
25:07 we we can proactively uh solve these problems and right then we know what to
25:13 expect and we can address them so and then having this data gives it all this power and then also with the dashboard
25:19 you know uh Sati knows what's happening as well and then getting that information to the customer showing the
25:26 troubleshooting and seeing that it's subjective because you know uh I can only imagine you know changing access
25:33 points or lowering the roof uh you know it's it's a very costly Endeavor you
25:38 know and you want to have it reliable right like what if we were wrong what if it was something else right then that
25:44 makes uh everyone look bad right uh but you know that's if you rely and you know
25:51 have proven metrics that um have been quickly valid or correctly validated
25:56 then we can avoid these issues so it's a great story yeah just just to add on
26:02 that n what you said is absolutely right because we need to make sure after we do the corrections after we fix the problem
26:08 right we need to see the results and we know that there are maybe another one or two sides we have something like that
26:15 but I was not confident hey I mean replacing AP is changing the the
26:20 building the structure the cabling it's not easy it's painful right and that's we have to depend on the remote remote
26:27 um site contact it was never easy and I was not confident until I see the data
26:33 from you okay after we fix all the issues now I was confident that okay we saw the Improvement in the zoom quality
26:40 overall experience right so that gave me confidence even going forward even if we
26:45 need to take this path for other sides we can do it now awesome um great to hear that the
26:53 clear communication was a big one and the success too um before I move over to the next section I
27:00 did want to put another disclaimer out there you know if you have any questions please put them the Q&A section here on
27:06 your screen um I believe we have one more trivia
27:12 question let me bring this up
27:19 and should be on your screen here so which breakthrough AI technology
27:25 achieved human level performance on a broad range of natural language understanding task in
27:31 2020 if you do not know this you might be under a rock
27:39 um I'll give a little
27:51 bit okay right it looks like majority answered at this point I'll go ahead and
27:58 share the results if you want to move over to the next slide if you answered
28:05 gpt3 you are correct um so if you've got all three of these right congrats three
28:11 gold stars for you um and we do appreciate your your
28:16 engagement so that being said let's keep
28:22 going so fatish now that we're towards kind of like the ending of the webinar
28:27 here um being a valued Juniper M AI customer one who have helped us um improve our AI
28:33 solution over the years uh what is the capability you like to see
28:39 next sure um since the M Marvis has air
28:45 engine it knows what's happening right it has a full data the complete visibility into the network and it also
28:52 kind of predicts some of the stuff right so I'm looking forward for the more some of of the the selfhealing capabilities
28:59 in the network level right it does maybe some little bit on the wireless probably
29:04 it's good to if we can expand further into the wireless I mean uh further more
29:09 from the wireless right switching and also the um the van Network and also
29:15 giving the proactive notification I'll give some of the scenarios for example if there is the issue with one of the
29:22 van circuit right not hard down but maybe the Laten issues or uh um maybe
29:28 the Jitter some of this the bandwidth concerns things like that so maybe this uh self healing um capabilities should
29:36 automatically fail over to another circuit right without doing any manual
29:42 intervention and also some of the proactiveness so since we have all the
29:47 data maybe it should predict some of the issues what's coming for example if the
29:53 user is have a scheduled meeting in one of the flo floor and um if Marvis says
30:00 there is some degradation in the network in that floor maybe should notify the US
30:05 user proactively saying that hey there is some uh Network issue kind of
30:11 probably and we we know that you have a schedule meeting coming up in this maybe a a side of the building maybe it's
30:19 better off you move other side of the building to different conference room so that way this kind of bringing the
30:25 proactiveness and the self filling identify the issues and proactively fix
30:30 that without even network engineer or operators being involved yeah so the
30:36 some of the things I'll be looking forward to those are all excellent ideas uh for
30:44 like I mentioned we have all the data now and you know I would actually just like to applaud service now and Satish
30:50 because they provided the data you know they trusted us with their data their data is secure in the cloud they did it
30:58 before Juniper did it right so we are able to uh have the data and play with
31:03 this before and it's just a real tribute they they you know they they you Sati
31:09 told us you know there's a problem here where we're having poor Zoom performance right and Juniper listened and uh the
31:17 Juniper Miss team basically hired me to solve uh this problem so and uh you know
31:24 just making the data available because you know we are not the the first step
31:29 you know just to Selena's first question you we we had the data because we had
31:35 the data we could do it and then uh we could solve these problems so and then you know all those things you mentioned
31:42 uh I was just noticing we have data points that can tell us that right so we can add them to the model so having this
31:48 feedback is um you know essential so please keep it going because you know we
31:54 test it and then we see does it actually solve the problem so um I'm looking forward to uh version two of the model
32:03 to to account for these things as
32:08 well um well thank you Sati for sharing that with us again we are working
32:13 towards that um so now that we're kind of towards the end of the webinar looks
32:19 like we have a few questions here um if you do have any questions again please
32:25 pop them into the Q&A um section there the first question we have is how do you
32:32 determine the efficacy of AI um and Satia I'm gonna actually
32:38 direct that one to you yeah
32:43 um the efficacy of AI right so we we heavily um depend on Marvis right even
32:50 at service now we have our own AI model but we're trying to not reinvent the whe
32:57 so use use the data what Marvis says and um on top of that we try to build our
33:03 own AI model right so what that mean so Maris um some of the times it provides
33:12 OT very good information right very good insights and we take the data right even we convert some of the data into a
33:19 service note ticket so that way we don't just see the data we take an action to
33:24 that so with some of the Integrations the direct integration with the Juniper mist and the service now so we
33:31 have that capability whatever Marv actions say that so we convert into service no tiate so we look into that
33:38 action hey is Marv's action telling right or do we see any discrepancy right
33:44 and uh because we validate I mean it it's good it is right most of the time but sometimes it is not getting the uh
33:51 right information that is where we provide the feedback to the Juniper team hey Marv is is telling maybe this bad
33:58 cable maybe uh two out of 10 cables maybe not the real bad cables right so
34:03 we give this data back to the m team so they run uh this data they evaluate and
34:10 come back with the feedback and try to solve this so this is kind of more back and forth like share we see the data
34:16 review the review the data and we share the feedback to J per Miss so they help
34:22 us to solve this I think it's kind of a journey I don't there's no clearcut um
34:27 step and it's a model right we have to train we have to train so we have the
34:32 data we have we got to we got to continuously um train it and improve improve the
34:40 product um we have another one here that says how long does it take to develop an
34:47 AI feature um I'm gonna go ahead throw that one at you nav you it's a really
34:53 good question so um I mean the the hard part is actually do we have the data and
35:01 then getting the data um it usually takes uh it can take sometimes if we
35:06 have the data for example um you know the schedule for a zoom call and if it's
35:11 uh occurs all the time um I imagine that data is there so we could have it and
35:17 then we could train the model so but then you know uh we add the feature in
35:22 to the model and then we test it has it improved it does it show up so and that's actually um going back to the
35:29 first question that was asked the efficacy right is it able to point it out or is it able to stand out where it
35:36 should right so the these are the sorts of things that we test so first getting the data and actually seeing if it's a
35:42 useful uh feature and does it show up when we think it will show up so um the
35:48 the whole process actually can take you know maybe about two to three weeks to do the whole uh data Gathering and uh
35:58 validation aome um and then I think we have room for one more uh so we have another that
36:06 says what's an example of an AI feature of the Miss solution that you and your team use daily and how has this improved
36:14 your operations so te I'm G give this one to
36:20 you um sure yeah so let me let me think about so in Marvis what do we use um
36:28 more frequently right Marvis actions are definitely a good one so we um Maris
36:35 actions and mql even I personally like the mql so I with the mql Maris sarey
36:42 language uh we can get the data very good actually I mean it has a data then if you need to generate some of the
36:48 report and it's very very uh useful and SL is the most important I I
36:55 look at that every day morning how is how is our Network looks like right
37:00 before my day starts just a few click the dashboard go there sles AR level
37:06 sles it tells you like how overall Wireless wide van the health status
37:12 across all the sides globally right that one and um Marvis actions is another
37:19 important one like as I was explaining before we heavily uh rely on the Marvis
37:27 because Marvis actions if if Marvis is telling something wrong with the DHCP we we really wanted to know what the
37:33 problem is right is it because of the connectivity issues or is it because of the actual DHCP server itself right or
37:40 it can be a DNS issue so some of these things uh we uh heavily rely on this and
37:45 as as I was briefing earlier we have the Integrations done with service now so
37:52 every Marvis action we take it serious and we try to review the data and if
37:58 there is any discrepancies we try to correct and by working with the Juniper
38:03 Miss team Marvis actions mql slus are the
38:09 most uh daily used features though thank you for sharing um I
38:17 believe that is it we have time for for Q&A I do want to share some resources um
38:24 with those who are interested uh so if you want to either whip out your phone now and just scan away or you can take a
38:31 screenshot I encourage that as well um and that way it's already saved to your desktop and you can bring it up at another time um but I encourage you to
38:38 read more on um the Gartner report we do have a few demos here and a few um or a
38:44 web page here of explainable in AI um and yeah we would love to have you on a
38:50 future demo if you are interested and that is all for now I do want to say
38:55 thank you to SA for joining us as well as navraj um we
39:01 do appreciate you all's uh engagement conversation wisdom all that good stuff
39:06 and again thank you again to the participants for your engagement in the trivia and hopefully you feel good about those three stars um and yeah that is
39:14 everything we thank you and hope to see you on the next one bye for
39:25 now